RSI, Relative Strength Index は、相場の過熱感を 0% - 100% で表したオシレーター系のテクニカル指標です。J・ウエルズ・ワイルダー・ジュニア氏 (J. Welles Wilder, Jr.) が考案者で、1978年に発表されました。日本語では、「相対力指数」と呼ばれます。
RSI の数値が 70% 以上では「買われすぎ」を意味し、30% 以下では「売られすぎ」を意味します。
算出方法は
RSI = 値上がり幅の指数移動平均(α) ÷ (値上がり幅の指数移動平均(α) + 値下がり幅の指数移動平均(α)) × 100
α = 1/14 を使うことをワイルダーは推奨しています。つまり、14 日の修正移動平均です。
先月 8 月 5 日の東京市場の歴史的な暴落を経験して、今さらながら先達が開発したテクニカル分析の手法を、自分でもプログラムを作って分析できるようにしようと思い、いろいろ調べています。
今回は上記 RSI です。
下記の OS 環境で動作確認をしています。
![]() |
RHEL 9.4 | x86_64 |
Python | 3.12.1 | |
jupyterlab | 4.2.5 | |
matplotlib | 3.9.2 | |
mplfinance | 0.12.10b0 | |
pandas | 2.2.2 | |
yfinance | 0.2.43 |
以下の作業は JupyterLab 上でおこなっています。
まず、必要なパッケージをインポートします。
import matplotlib.pyplot as plt import mplfinance as mpf import pandas as pd import yfinance as yf |
Wilder の RSI を算出する関数です。参考サイト [1] で公開されている Python のコードを流用させていただきました。
def wilder_rsi(ohlc: pd.DataFrame, period: int = 14 ) - > pd.Series: delta = ohlc[ 'Close' ].diff() up, down = delta.copy(), delta.copy() up[up < 0 ] = 0 down[down > 0 ] = 0 _gain = up.ewm(com = (period - 1 ), min_periods = period).mean() _loss = down. abs ().ewm(com = (period - 1 ), min_periods = period).mean() rs = _gain / _loss return pd.Series( 100 - ( 100 / ( 1 + rs)), name = 'RSI' ) |
過去1年分の日経平均株価 (^N225) の日足データを取得します。
symbol = '^N225' ticker = yf.Ticker(symbol) df = ticker.history(period = '1y' ) |
mplfinance でローソク足チャートとその移動平均(5 日, 25 日, 75 日)をプロットし、その下の二番目の領域に RSI をプロットします。チャートに手を加えたかったので mplfinance にプロットの全てを任せず、わざわざ matplotlib で扱えるようにしています。
# Wilder RSI rsi = wilder_rsi(df) fig, ax = plt.subplots( 2 , 1 , gridspec_kw = dict (height_ratios = [ 2 , 1 ], hspace = 0 ), sharex = True , figsize = ( 12 , 6 ) ) apd_osc = [ mpf.make_addplot(rsi, ylabel = 'RSI' , color = 'black' , width = 0.5 , ax = ax[ 1 ]) ] mpf.plot( df, type = 'candle' , style = 'yahoo' , mav = ( 5 , 25 , 75 ), addplot = apd_osc, ax = ax[ 0 ], ) ax[ 0 ].set_title( '%s (%s)' % (ticker.info[ 'longName' ], symbol)) ax[ 0 ].grid( 'both' ) fb_rsi_up = dict (x = range ( len (rsi)), y1 = rsi.values, y2 = 50 , where = 50 <rsi, color = 'blue' , alpha = 0.25 , interpolate = True ) fb_rsi_dn = dict (x = range ( len (rsi)), y1 = rsi.values, y2 = 50 , where = 50 >rsi, color = 'red' , alpha = 0.25 , interpolate = True ) ax[ 1 ].fill_between( * * fb_rsi_up) ax[ 1 ].fill_between( * * fb_rsi_dn) ax[ 1 ].set_ylabel( 'Wilder RSI' ) ax[ 1 ].grid( 'both' ) ax2 = ax[ 1 ].twinx() ax2.set_ylim(ax[ 1 ].get_ylim()) ax2.tick_params(axis = 'y' ) ax2.set_yticks([ 30 , 50 , 70 ]) yticklabels2 = ax2.get_yticklabels() yticklabels2[ 0 ].set_color( 'red' ) yticklabels2[ 1 ].set_color( 'black' ) yticklabels2[ 2 ].set_color( 'blue' ) ax2.axhline(y = 30 , color = 'red' , linewidth = 0.75 , linestyle = 'solid' ) ax2.axhline(y = 50 , color = 'black' , linewidth = 0.75 , linestyle = 'solid' ) ax2.axhline(y = 70 , color = 'blue' , linewidth = 0.75 , linestyle = 'solid' ) plt.subplots_adjust(left = 0.15 , right = 0.85 , bottom = 0.05 , top = 0.95 ) # plt.savefig('rsi_wilder_n225.png') plt.show() |
RSI をただプロットするだけでは味気ないので、(実用的かどうかは別として)いろいろ修飾してみました。💦
参考サイト
- RSI calculation to match Tradingview
- RSI には2つの計算式があるけれど違いは何か [2023-09-26]
- bitWalk's: 【備忘録】モメンタムとボリンジャーバンド [2024-08-10]
- bitWalk's: 【備忘録】フィボナッチ・リトレースメント [2024-08-12]
- bitWalk's: 【備忘録】一目均衡表 [2024-08-15]

にほんブログ村
#オープンソース
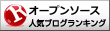
0 件のコメント:
コメントを投稿