scikit-learn は、Python のオープンソース機械学習ライブラリです。SVM, Random Forest, Gradient Boosting, k平均法、DBSCAN などを含む様々な分類、回帰、クラスタリングアルゴリズムを備えており、Python の数値計算ライブラリの NumPy, SciPy とやり取りするよう設計されています。
Python で機械学習を扱うとき、いや、主に主成分分析 (PCA) でデータを整理するときにと、言い直した方が良いような気がするのですが、そんな時に利用するライブラリは scikit-learn のほぼ一択になっています。
前処理でデータを標準化をするときには StandardScaler、すなわち平均値と標準偏差でデータを標準化するスケーラーで十分だと思っていたのですが、よくよく考えてみると、決してそうではないことに気づきました。だとすれば、スケーラーをカスタマイズしたくなりますが、StandardScaler のソースを見て、これをベースにカスタマイズすることを諦めてしまいました。😅
とりあえず、最低限の機能を実装した StandardScaler と同等機能の超シンプルなスケーラーを作って、それをベースにカスタマイズしていくことにしました。
ということで、下記の CustomScaler クラスは、自分の使う範囲では StanbdardScaler と同じ機能しかありませんが、これをベースにカスタムなスケーラを作っていくので、備忘録としました。ま、不具合が出れば StandardScaler のソースを見て手直しを重ねます。😁
import numpy as np | |
import pandas as pd | |
from sklearn.datasets import load_iris | |
from sklearn.decomposition import PCA | |
from sklearn.pipeline import Pipeline | |
from sklearn.preprocessing import StandardScaler | |
class CustomScaler: | |
"""Custome Scaler (template) | |
""" | |
def __init__(self): | |
pass | |
def fit(self, x: np.ndarray, *args): | |
# mean | |
self.mean_ = np.mean(x, axis=0) | |
# standard deviation | |
self.std_ = np.std(x, axis=0) | |
def transform(self, x: np.ndarray, *args) -> np.ndarray: | |
return (x - self.mean_) / self.std_ | |
def fit_transform(self, x: np.ndarray, *args) -> np.ndarray: | |
self.fit(x, *args) | |
return self.transform(x, *args) | |
if __name__ == '__main__': | |
ds = load_iris() | |
features = ds['data'] | |
# Standard Scaler | |
pipe_standard = Pipeline( | |
steps=[('scaler', StandardScaler()), | |
('PCA', PCA())] | |
) | |
components_standard = pipe_standard.fit_transform(features) | |
df_pca_normal = pd.DataFrame( | |
data=components_standard, | |
columns=['PC{}'.format(i + 1) for i in range(components_standard.shape[1])] | |
) | |
print('>>> Standard Scaler') | |
print(df_pca_normal.head()) | |
# Custom Scaler | |
pipe_custom = Pipeline( | |
steps=[('scaler', CustomScaler()), | |
('PCA', PCA())] | |
) | |
components_custom = pipe_custom.fit_transform(features) | |
df_pca_custom = pd.DataFrame( | |
data=components_custom, | |
columns=['PC{}'.format(i + 1) for i in range(components_custom.shape[1])] | |
) | |
print('>>> Custom Scaler') | |
print(df_pca_custom.head()) |
動作確認用に iris のデータセットを使って、StandardScaler と CustomScaler それぞれで標準化したあと PCA をして、結果をざっくり比較しています(結果が同じになるべきです)。
>>> Standard Scaler PC1 PC2 PC3 PC4 0 -2.264703 0.480027 -0.127706 -0.024168 1 -2.080961 -0.674134 -0.234609 -0.103007 2 -2.364229 -0.341908 0.044201 -0.028377 3 -2.299384 -0.597395 0.091290 0.065956 4 -2.389842 0.646835 0.015738 0.035923 >>> Custom Scaler PC1 PC2 PC3 PC4 0 -2.264703 0.480027 -0.127706 -0.024168 1 -2.080961 -0.674134 -0.234609 -0.103007 2 -2.364229 -0.341908 0.044201 -0.028377 3 -2.299384 -0.597395 0.091290 0.065956 4 -2.389842 0.646835 0.015738 0.035923
参考サイト
- sklearn.preprocessing.StandardScaler — scikit-learn 1.1.3 documentation
- sklearn.decomposition.PCA — scikit-learn 1.1.3 documentation
- sklearn.datasets.load_iris — scikit-learn 1.1.3 documentation

にほんブログ村
#オープンソース
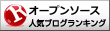
0 件のコメント:
コメントを投稿